Labeling Tools for Machine Learning: A Comprehensive Guide
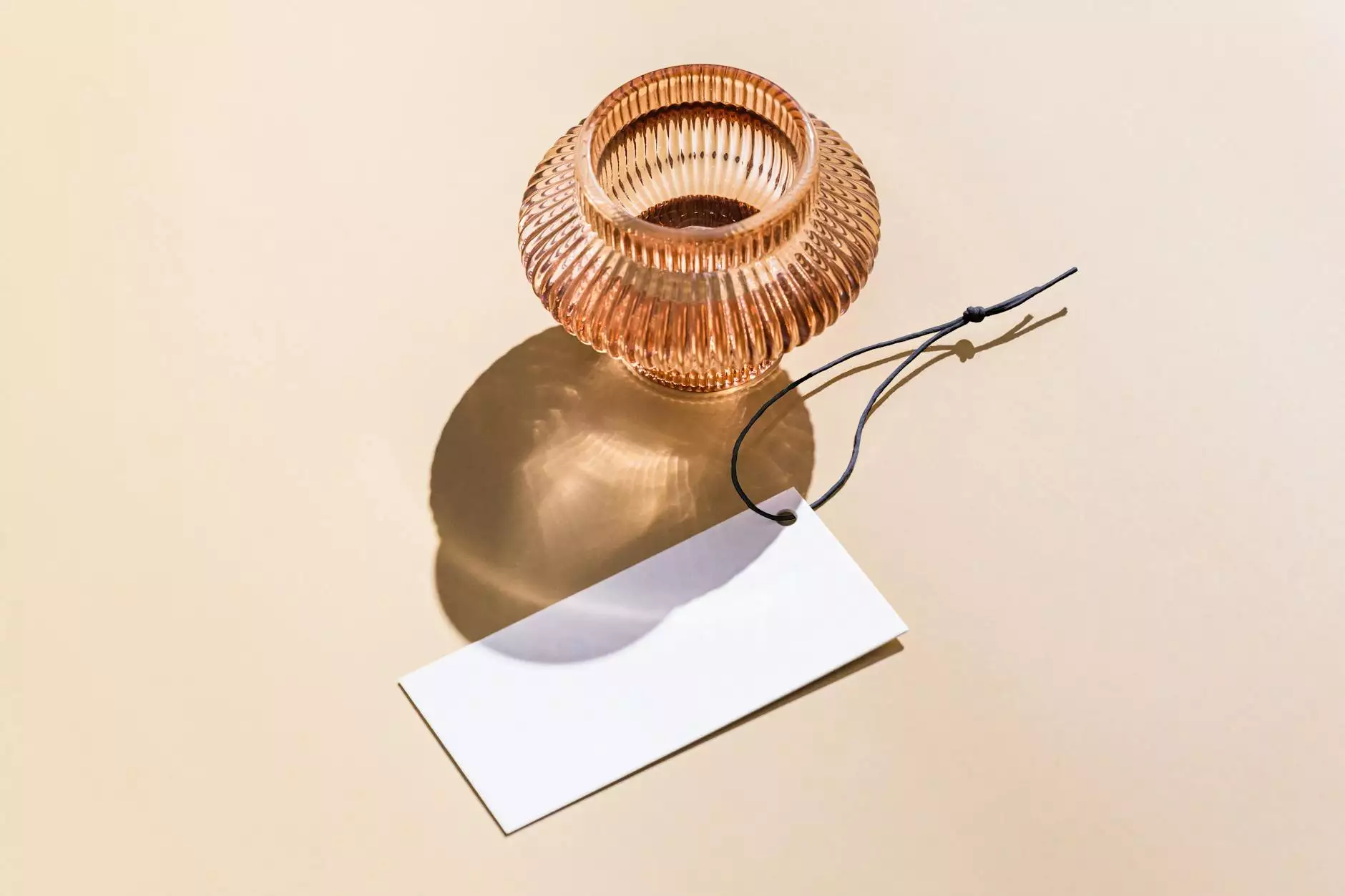
In the ever-evolving world of technology, machine learning stands out as a pivotal force driving innovation across various industries, including Home Services, Keys & Locksmiths, and more. One of the fundamental requirements of machine learning is the quality of data fed into algorithms. This is where labeling tools for machine learning come into play. Understanding what these tools are, their importance, and how they can revolutionize your business will provide you with a competitive edge in a data-driven environment.
Understanding Machine Learning and Data Labeling
Machine learning is a subset of artificial intelligence that enables computers to learn from data and make decisions. However, for machines to learn effectively, they need accurately labeled data. Data labeling is the process of annotating data to teach algorithms to recognize patterns. It involves tagging data points such as images, text, and audio with labels that indicate their characteristics or classifications.
What Are Labeling Tools?
Labeling tools for machine learning are software applications designed to streamline the data labeling process. These tools facilitate the annotation of datasets, ensuring that the data is correctly labeled to improve the accuracy and performance of machine learning models.
Importance of Labeling Tools in Machine Learning
- Accuracy and Precision: High-quality labeled data leads to better model performance. Labeling tools minimize human error and enhance the precision of data annotations.
- Efficiency: These tools automate parts of the labeling process, significantly reducing the time needed to prepare datasets.
- Collaboration: Many labeling tools support multiple users, making it easier for teams to collaborate on data labeling tasks.
- Scalability: As businesses grow, the volume of data increases. Labeling tools can scale up to handle massive datasets, ensuring continuous improvement in machine learning applications.
Types of Labeling Tools for Machine Learning
Labeling tools can be categorized into various types based on their functionalities and the data they support. Here are some of the most common types:
1. Image Annotation Tools
These tools are specifically designed for labeling images, which is particularly useful in fields such as autonomous driving, healthcare, and security. Common functionalities include:
- Bounding Box Annotation: Used to identify and categorize objects within an image.
- Segmentation: Divides an image into segments to identify different objects or areas.
- Keypoint Annotation: Marks specific points on an object, commonly used in pose estimation.
2. Text Annotation Tools
Text annotation tools allow users to tag parts of text data, beneficial for tasks like sentiment analysis, named entity recognition, and more:
- Entity Recognition: Identifies and classifies key information (names, locations, dates).
- Sentiment Annotation: Labels text based on its sentiment (positive, negative, neutral).
- Intent Recognition: Helps in assessing user intent in chatbots and virtual assistants.
3. Audio Annotation Tools
These tools provide functionalities to label audio data, crucial for applications in speech recognition and natural language processing (NLP):
- Transcription: Converts spoken language into text format.
- Speaker Identification: Tags who is speaking in multi-speaker environments.
- Emotion Detection: Analyzes audio to determine the emotional state of the speaker.
How to Choose the Right Labeling Tool for Your Business
Choosing the appropriate labeling tool for machine learning can be a daunting task. Here are some factors to consider:
1. Data Type
Select a tool that supports the specific type of data you will be labeling, whether it's images, text, or audio.
2. User Interface
A user-friendly interface is essential for efficiency. The easier it is to use, the faster your team can annotate data.
3. Collaboration Features
For businesses with multiple team members, collaboration features are crucial. Look for tools that allow multiple users to work simultaneously.
4. Scalability
Your labeling tool should be able to handle increasing amounts of data as your business grows.
5. Integration Capabilities
Consider how well the labeling tool integrates with your existing systems and workflows.
Popular Labeling Tools for Machine Learning
There are numerous labeling tools available in the market today. Some of the leading tools include:
1. Labelbox
Labelbox is a versatile labeling tool that supports image, video, and text data. Its collaborative interface and robust API make it a favorite among data scientists.
2. Supervisely
Supervisely is specifically geared towards image and video annotation, providing advanced features such as 3D point cloud annotation and neural network training.
3. Prodigy
Prodigy focuses on text annotation and is designed for machine learning practitioners. Its active learning capability ensures that you focus on the most informative examples.
4. Snorkel
Snorkel allows users to build and train labeling functions to automate the labeling process, making it particularly useful for large-scale projects.
5. VGG Image Annotator (VIA)
VIA is an open-source tool that supports image annotation tasks such as bounding boxes, points, and polygons. It’s lightweight and requires no installation, making it accessible for quick projects.
Best Practices for Data Labeling
When utilizing labeling tools for machine learning, it’s essential to follow best practices to ensure data quality:
1. Clear Guidelines
Develop clear labeling guidelines to ensure consistency across your dataset. This is crucial when multiple annotators are involved.
2. Quality Assurance
Implement a quality assurance process wherein a subset of labeled data is reviewed to ensure accuracy and precision.
3. Continuous Feedback
Encourage annotators to provide feedback on the labeling process. This can help identify any ambiguities or difficulties they face.
4. Annotation Audit
Conduct regular audits of labeled data to maintain high standards and rectify any inaccuracies that may arise.
The Future of Labeling Tools in Machine Learning
As machine learning continues to advance, labeling tools for machine learning are also evolving. Here are some trends shaping their future:
1. Automation and AI Integration
With advancements in AI, we can expect more automation in the labeling process. Tools that leverage AI to assist human annotators will increase efficiency.
2. Enhanced Collaboration Features
As remote work becomes more commonplace, tools that enable seamless collaboration among team members, regardless of location, will be paramount.
3. Real-Time Analytics
Future labeling tools will likely offer real-time analytics, allowing teams to monitor progress and quality as data is being labeled.
Conclusion
Incorporating the right labeling tools for machine learning into your business strategy can vastly enhance the efficiency and accuracy of your machine learning projects. Whether you operate in Home Services, Keys & Locksmiths, or any other industry, understanding the importance and utility of data labeling is critical. With the right tools and practices, you can unlock the full potential of your data and stay ahead in the competitive landscape of technology-driven business solutions.
Explore the resources available at keymakr.com to further delve into how machine learning and its associated tools can transform your operations and drive growth.